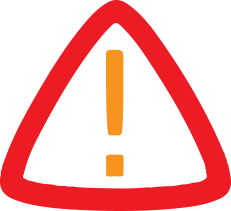
New Study Reveals Widespread Underestimation Of Income Of The Richest
The research, led by Barnabás Szászi, examined data from over 2,700 participants in a series of experiments. Across all four studies, participants were found to significantly underestimate the resources held by the top 1% of earners, compared to more accurate estimates for lower-income groups.
“Our findings highlight a fundamental cognitive bias-scope insensitivity-that prevents people from accurately perceiving just how concentrated income is at the top,” explains Szászi.“This misperception likely contributes to the lower support for redistribution, despite rising inequality.”
The study demonstrates that while people are often aware of inequality, they do not fully grasp the magnitude of income concentrated among the richest individuals. The researchers argue that addressing these cognitive biases is critical to increasing public awareness of income disparities and developing more effective policies to tackle inequality.
Key Findings of the Study:
People consistently underestimate the income thresholds of the top 1% compared to more accurate perceptions of other income brackets.
This selective underestimation may be driven by cognitive biases like scope insensitivity, where large numbers (such as top incomes) become harder to comprehend.
Correcting these misperceptions could be challenging, as systemic factors like media representation and geographic segregation reinforce them.
With inequality rising sharply in developed countries, this study provides valuable insights for policymakers looking to address the public's understanding of income distribution.
For further information, please visit the study page on PNAS Nexus or contact Barnabás Szászi at the Institute of Psychology, Eötvös Loránd University, Budapest.
Barnabas Imre Szaszi
Eotvos Lorand University (ELTE)
+36 30 565 3943
email us here
Visit us on social media:
X
LinkedIn
Legal Disclaimer:
EIN Presswire provides this news content "as is" without warranty of any kind. We do not accept any responsibility or liability for the accuracy, content, images, videos, licenses, completeness, legality, or reliability of the information contained in this article. If you have any complaints or copyright issues related to this article, kindly contact the author above.
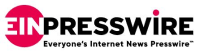
Legal Disclaimer:
MENAFN provides the information “as is” without warranty of any kind. We do not accept any responsibility or liability for the accuracy, content, images, videos, licenses, completeness, legality, or reliability of the information contained in this article. If you have any complaints or copyright issues related to this article, kindly contact the provider above.
Comments
No comment